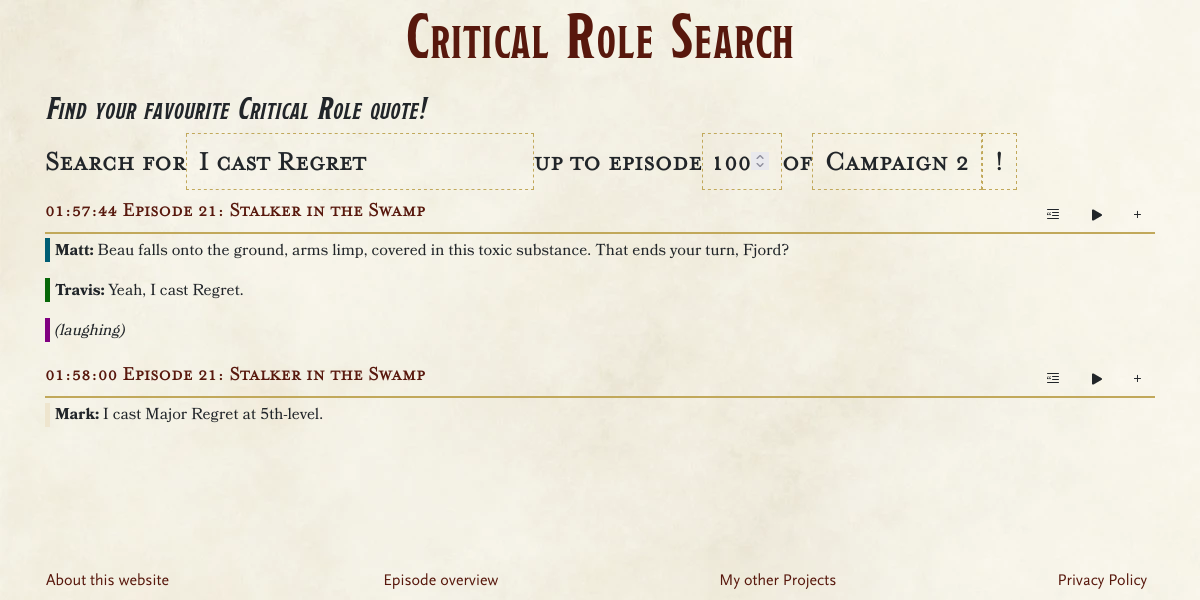
Critical Role Search
Find your favourite Critical Role quote!
Critical Role is an online series on Twitch and YouTube where a group of voice actors plays Dungeons & Dragons. With over 300 episodes and more than 1400 hours of gameplay there is a huge amount of existing content which makes looking up a quote and finding the corresponding part of the video where it occurred. Thankfully volunteers transcribed the first 169 episodes and since then official subtitles are provided by the Critical Role team. This means that all together we got a dataset of nearly 12 million words we can search through.
My Critical Role Search can do this efficiently while respecting the user-specified episode limit to avoid showing spoilers. Lines of dialogue are color-coded for every player and more context or a video-player at this exact timestamp can be displayed for every search result. In addition, the search field can auto-complete every phrase
See also:
- An overview over every video that is imported in the database
- Some statistics calculated from the dataset
- An experimental transcript view
Also check out www.kryogenix.org/crsearch which is a very similar website by Stuart Langridge that I found just after publishing my website.
Technical Details
I wanted to make sure that the search feels as instant as possible. So both search and auto-complete should return
results for a smaller number of episodes in around 50ms and still load reasonably fast (less than 1 second) even when
searching through all 100+ episodes of one campaign. After loading the subtitles from YouTube, a large part of the
complexity of the project is transforming the lines of
subtitles into full sentences assigned to the corresponding speakers (while keeping the timestamps intact). The
information about each episode and line is then stored in a PostgreSQL database. Then
PostgreSQL full text search is used for the actual search.
For this, in addition to the actual text, a tsvector
containing the text converted into tokens and lexemes (so the
normalized form) like 'exampl':5 'longer':4 'text':6
.
INSERT INTO line (text, search_text, ...)
values ('This is a longer example text', to_tsvector('english', 'This is a longer example text'));
Now the search query only needs to be converted into a tsquery
like 'cast' & 'regret'
and we can look up a query
extremely quickly with a query like this:
SELECT *, ts_rank("search_text", websearch_to_tsquery('english', 'I cast regret')) AS rank
FROM line
INNER JOIN person ON (line.person_id = person.id)
INNER JOIN episode ON (line.episode_id = episode.id)
WHERE (
(line.search_text @@ websearch_to_tsquery('english', 'I cast regret')) AND
(episode.episode_number <= 1000) AND
(episode.series_id = 2)
)
ORDER BY rank DESC
LIMIT 20;
This has a few flaws (the ranking by ts_rank
is very basic, substrings sometimes have priority over the full search
query, etc.), but works easily without needing any other database just for the search.
For the search auto-complete I wanted to suggest not only popular phrases, but every single phrase that occurs anywhere while still avoiding spoilers and prioritising common phrases. Therefore, I used the spaCy Natural Language Processing Toolkit to extract noun chunks (like a longer example text
) from all lines and aggregate their count.
SELECT *
FROM phrase
join episode e on e.id = phrase.episode_id
WHERE episode_number = 1
and series_id = 2
ORDER BY count DESC
-- displaying a random sample below
+------+-------------------+-----+----------+
|id |text |count|episode_id|
+------+-------------------+-----+----------+
|316913|They |143 |119 |
|317032|what |121 |119 |
|316899|you guys |35 |119 |
|317883|The tent |31 |119 |
|317006|people |28 |119 |
|316927|the show |23 |119 |
|318966|your turn |18 |119 |
|317811|Yasha |16 |119 |
|317319|the Crown's Guard |12 |119 |
|317531|the room |12 |119 |
|317938|Frumpkin |11 |119 |
|317510|the front |11 |119 |
|318356|place |10 |119 |
|317424|this point |10 |119 |
|317396|Nott |10 |119 |
|317667|a perception check |8 |119 |
+------+-------------------+-----+----------+
By aggregating these over all episodes and filtering phrases that contain the query word, we get a ranked list of search suggestions:
SELECT phrase.text, SUM(phrase.count) AS total_count
FROM phrase
INNER JOIN episode ON (phrase.episode_id = episode.id)
WHERE episode.series_id = 2
AND episode.episode_number <= 10
AND phrase.text ILIKE '%dama%'
GROUP BY phrase.text
ORDER BY total_count DESC
LIMIT 10;
+------------------+-----------+
|text |total_count|
+------------------+-----------+
|damage |221 |
|piercing damage |42 |
|Roll damage |27 |
|bludgeoning damage|18 |
|poison damage |13 |
|cold damage |10 |
|the damage |10 |
|Port Damali |9 |
|psychic damage |8 |
|fire damage |6 |
+------------------+-----------+
As %query%
LIKE
queries are quite slow, we can speed them up significantly by adding a gin
index to the table:
CREATE INDEX phrases_text_index ON phrase USING gin (text gin_trgm_ops)